AI analytics is transforming how businesses make decisions by leveraging vast amounts of data. It involves collecting and processing data, applying machine learning models, and visualizing results.
In this post, we at Emplibot will explore key components, practical applications, and both the advantages and limitations of AI analytics.
Stay informed on how AI analytics can boost efficiency across various industries and see what the future holds for this dynamic field.
Contents
ToggleWhat Are Key Components?
Gathering and Cleaning Data
The first step is gathering and cleaning data, an often underestimated but vital process. On average, data scientists spend about 80% of their time on cleaning data. This step involves removing duplicates, correcting errors, and filling in missing values to create a high-quality dataset. The quality of your data directly impacts the performance of your machine learning models.
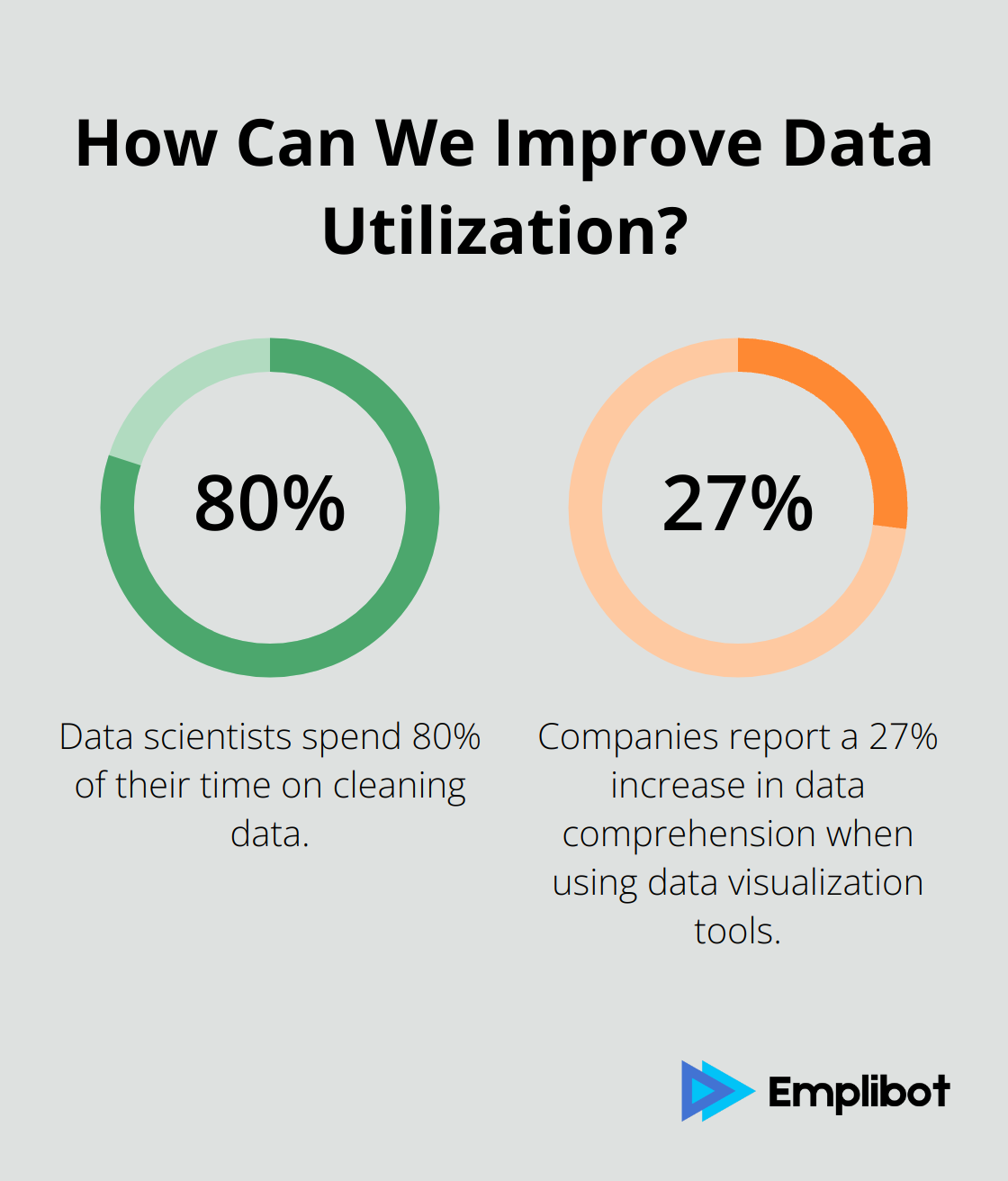
For tools, consider using open-source options like Pandas for data cleaning and Apache Spark for processing large datasets efficiently. These tools help manage vast amounts of data swiftly and ensure accuracy.
Implementing Machine Learning Models
Once your data is clean, it’s time to implement machine learning models and algorithms. Machine learning models thrive on quality data. Algorithms such as decision trees, neural networks, and support vector machines each serve different purposes. For example, decision trees are ideal for classification tasks, whereas neural networks excel in recognizing complex patterns, crucial for image recognition tasks.
Practical resources for implementing these models include scikit-learn and TensorFlow. These platforms offer extensive libraries and pre-built functions, making it easier to train models and fine-tune parameters. The key is knowing which model suits your specific needs, as selecting the wrong algorithm can lead to inaccurate predictions, wasting resources.
Visualizing Results
Visualizing results is essential for interpreting machine learning outputs and making data-driven decisions. Visualization tools like Tableau and Power BI allow you to create interactive dashboards. These tools transform complex data into understandable visuals.
According to a survey by Business Application Research Center (BARC), companies that use data visualization tools report a 27% increase in data comprehension across teams. Interactive visuals make it easier to spot trends, identify outliers, and share insights with stakeholders.
By leveraging visualization tools, businesses can translate intricate datasets into actionable insights, drive better decision-making, and ultimately enhance operational efficiency.
For further insights into using AI for visualization, you might find our guide on AI-powered image recognition beneficial.
What Are Applications?
AI analytics is revolutionizing various industries through its practical applications. Here, we focus on specific use cases in manufacturing, retail, and finance to highlight how AI analytics can drive efficiency and innovation.
Predictive Maintenance in Manufacturing
Predictive maintenance is a game-changer for the manufacturing sector. Instead of relying on scheduled maintenance, predictive algorithms analyze data from sensors and machinery to predict when equipment is likely to fail. According to Deloitte, predictive maintenance can reduce breakdowns by up to 70% and lower maintenance costs by 25%.
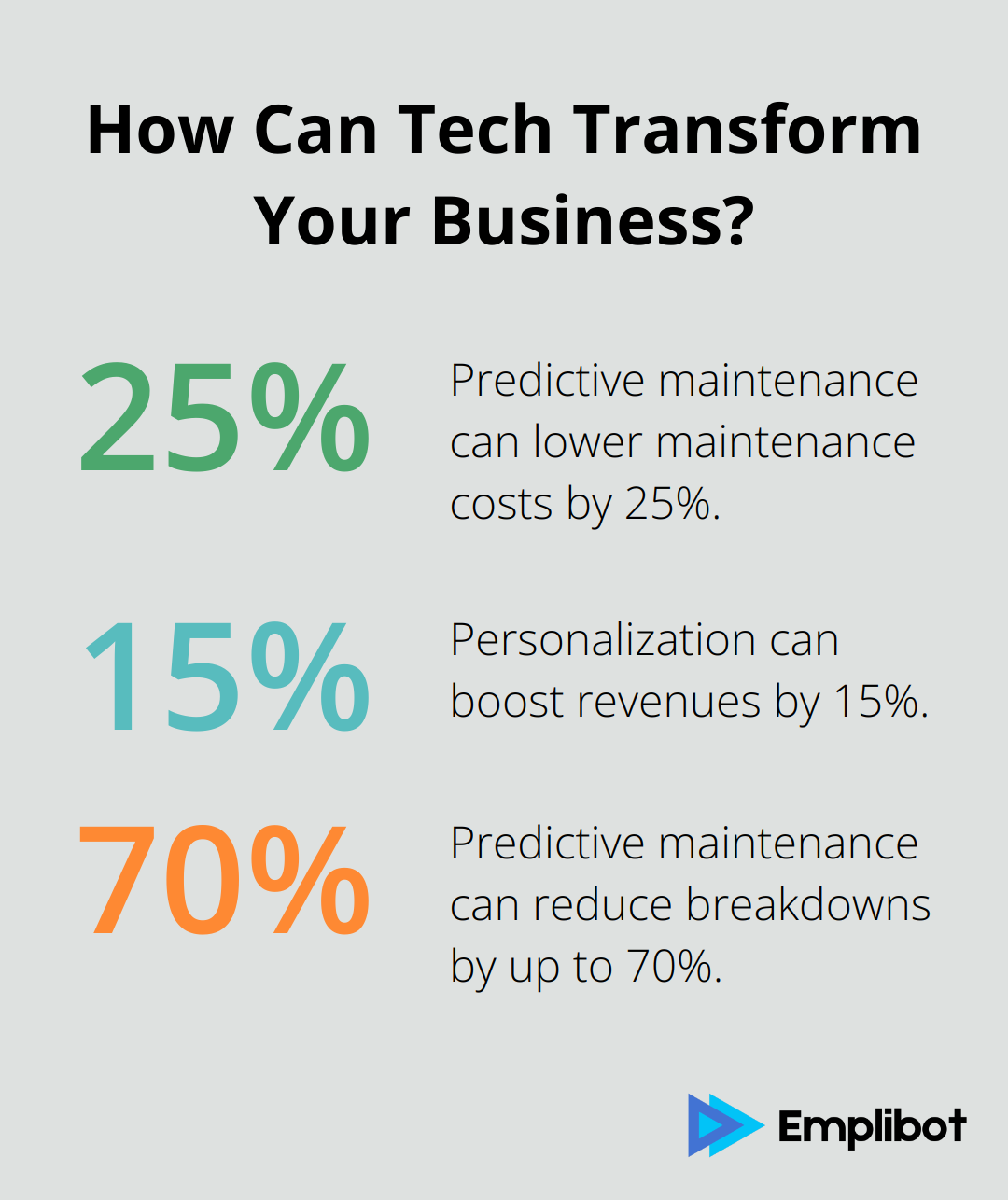
For implementation, integrating AI with Internet of Things (IoT) devices provides real-time data. Tools like Predix by GE Digital help in monitoring machinery health and forecasting potential issues. Companies like Siemens have successfully used AI to shift from reactive to predictive maintenance, enhancing operational efficiency and significantly reducing downtime.
Personalized Marketing in Retail
Retailers leveraging AI analytics for personalized marketing see remarkable results. Personalization can boost revenues by 15% according to McKinsey. AI analyzes customer data to predict shopping behaviors and preferences, enabling tailored marketing strategies.
Use AI-powered tools such as Amazon Personalize and Salesforce Einstein to create targeted campaigns. A case in point is Sephora, which uses AI to provide personalized product recommendations, increasing customer engagement and sales. By analyzing browsing history, purchase patterns, and feedback, AI engines deliver highly relevant suggestions, improving the shopping experience.
For practical insights, see our guide on personalized marketing with AI.
Fraud Detection in Finance
Fraud detection in finance has been exponentially improved by AI analytics. Financial institutions can now detect fraudulent activities in real-time with high accuracy. According to PwC, AI can reduce fraud detection costs by 20% and improve detection rates by 50%.
Adopt advanced AI models like neural networks and anomaly detection algorithms, which can sift through vast amounts of data to identify unusual patterns. Companies like PayPal use AI to analyze transaction data and identify fraudulent behaviors, thus protecting customers and minimizing financial losses.
Using tools like SAS Fraud Management or ThreatMetrix can also be highly effective for financial firms looking to enhance their security measures.
By implementing these AI analytics applications, industries can realize significant gains in efficiency, customer satisfaction, and security. As AI technologies continue to evolve, the potential for transformative impact across various sectors will only increase.
What Are the Advantages and Limitations of AI Analytics?
Improved Decision-Making and Efficiency
AI analytics significantly boosts decision-making and operational efficiency across industries. According to McKinsey, businesses employing AI analytics see a 6% to 9% improvement in operational performance. By automating data analysis, AI frees up human resources for strategic tasks, enabling faster and more informed decisions. For instance, eCommerce businesses using AI analytics can detect and correct conversion issues in real-time, averting potential revenue losses.
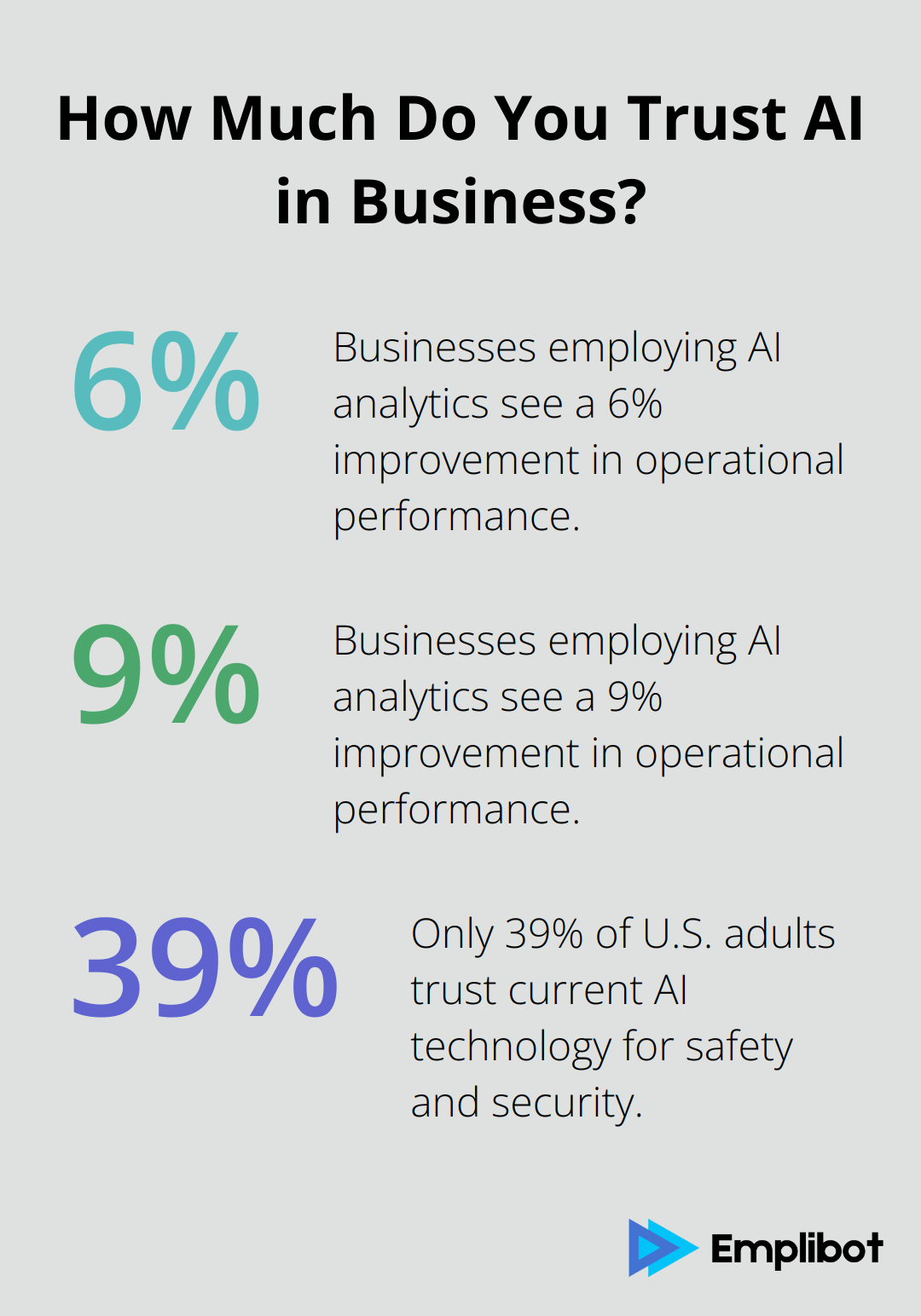
Additionally, AI’s predictive capabilities allow companies to anticipate customer needs and market trends, giving them a competitive edge. It’s evident in the retail sector where personalized marketing, driven by AI, leads to a 15% rise in revenues.
Scalability and Real-Time Analysis
AI analytics excels in scalability and real-time data processing. Traditional analytics often struggle with large datasets, but AI scales effortlessly, processing terabytes of data swiftly. Tools like Apache Kafka enable the real-time handling of streaming data, critical for industries relying on immediate insights, such as finance and healthcare.
Furthermore, real-time AI analysis enhances adaptive strategies. For example, AI-powered marketing dashboards offer instant feedback on campaign performance, allowing marketers to tweak strategies on the fly. This adaptability is why 73% of U.S. companies use AI in some capacity.
Data Privacy and Accuracy Challenges
Despite the benefits, AI analytics brings challenges, particularly around data privacy and accuracy. A survey by the Pew Research Center found that only 39% of U.S. adults trust current AI technology for safety and security. Issues like data breaches and lack of transparency in AI decision-making processes exacerbate these concerns.
Accuracy is another significant hurdle. AI systems are only as good as the data they are trained on. According to a study by IBM, poor data quality costs businesses $3.1 trillion annually in the U.S. alone. Ensuring data integrity, therefore, is paramount. Errors in data input or processing can lead to faulty AI predictions, making data governance a critical responsibility.
Incorporating comprehensive data management practices and robust encryption tools can address these challenges. Organizations must prioritize ethical AI practices to balance efficiency with privacy considerations. For deeper insights on managing AI’s ethical implications, you might find our guide on ethical AI marketing valuable.
Conclusion
AI analytics encompasses crucial processes like data gathering and cleaning, the application of machine learning models, and result visualization. Each step is integral to achieving accurate and actionable insights. Businesses across diverse sectors, from manufacturing to retail and finance, are realizing substantial benefits through applications such as predictive maintenance, personalized marketing, and fraud detection.
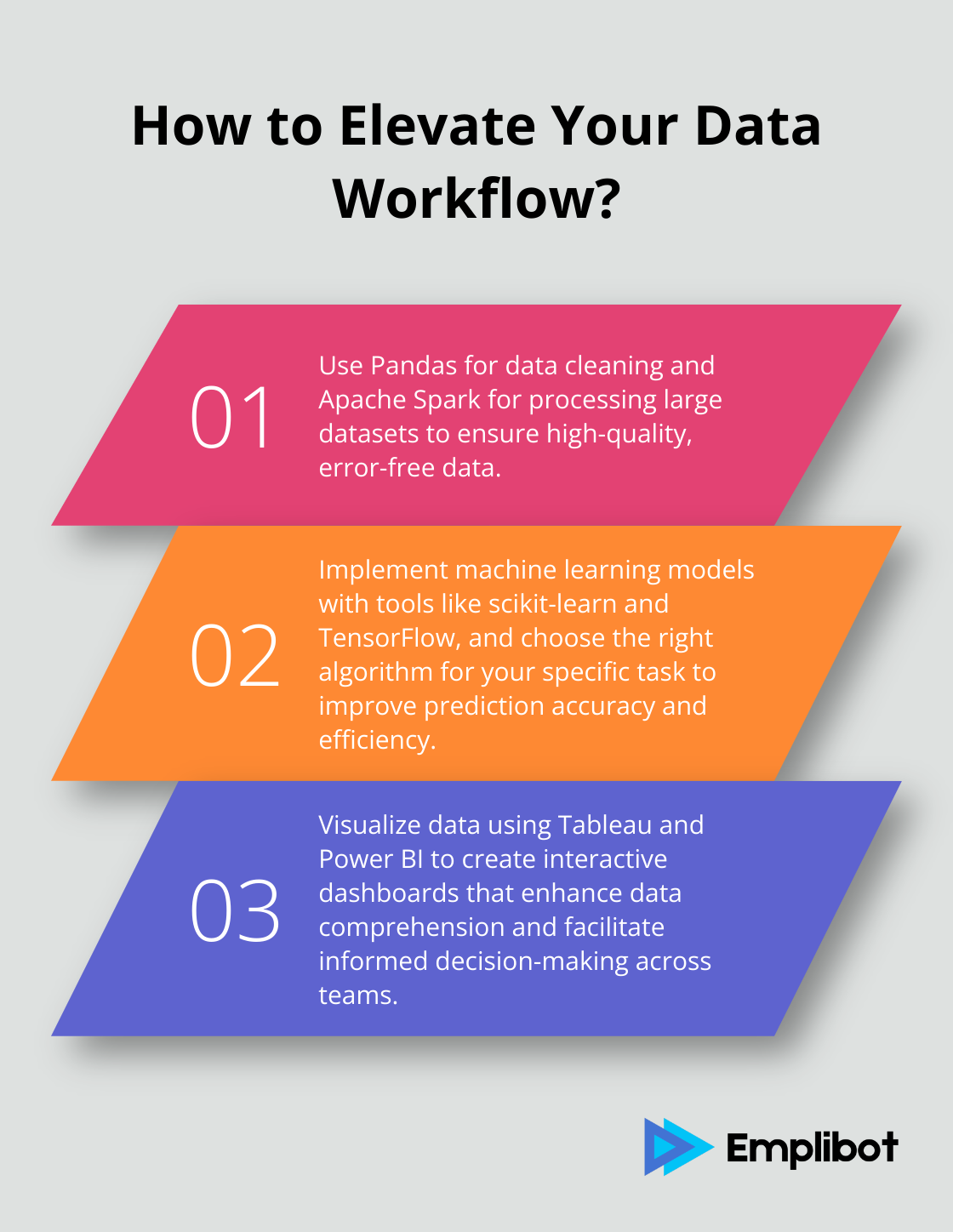
Looking ahead, AI analytics is poised to drive further advancements in operational efficiency, scalability, and real-time data analysis. As organizations continue to prioritize AI integration, they will need to navigate challenges related to data privacy and accuracy. Enhancing data governance practices and adopting ethical AI frameworks will be essential.
Embracing AI analytics offers a significant competitive edge by fostering better decision-making, increased adaptability, and proactive strategies. We at Emplibot are committed to supporting businesses in leveraging AI to its full potential. For those seeking to enhance their content strategy with AI automation, explore Emplibot for comprehensive solutions. As AI technologies evolve, the transformative impact on industries will only deepen, making this an opportune time to invest in AI analytics.
For further insights, consider reading about real-time analytics or AI sentiment analysis.