AI-powered image recognition is transforming the way we interact with technology, offering revolutionary capabilities across countless industries. We at Emplibot are excited to share insights into the core principles and applications that are paving the way for this innovation.
Best practices are pivotal in harnessing the full potential of AI in image analysis. This post is designed to guide you through implementing, optimizing, and maximizing the technology for enhanced accuracy and efficiency.
Contents
ToggleMastering AI Image Recognition
Navigating the complexities of AI-powered image recognition requires a nuanced understanding of not only the technology but also the strategy behind successful implementation. It’s not about deploying the most sophisticated tools, but about optimizing those tools to work flawlessly with your objectives. Practicality and purpose should be the driving forces behind your image recognition efforts. Here’s how you can amplify your approach to AI image analysis for outstanding results.
Essential Building Blocks of Image Recognition
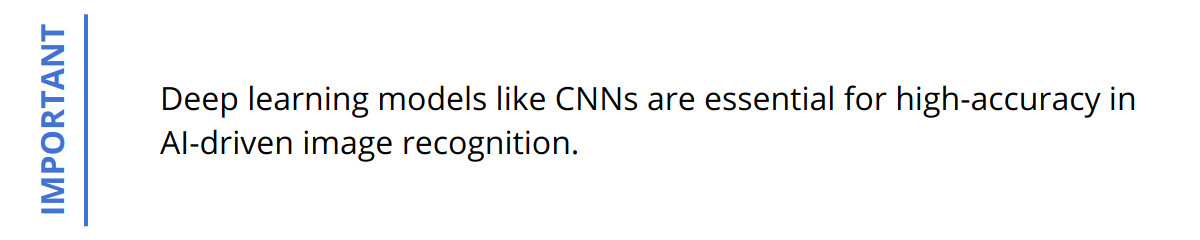
At the core of AI-driven image recognition are deep learning models, notably Convolutional Neural Networks (CNNs), that have revolutionized accuracy in classifying and identifying patterns in images. These models thrive on high-quality, annotated datasets. With sufficient training, they can distinguish between objects with a level of precision that was previously unattainable.
Leveraging AI for Enhanced Image Analysis
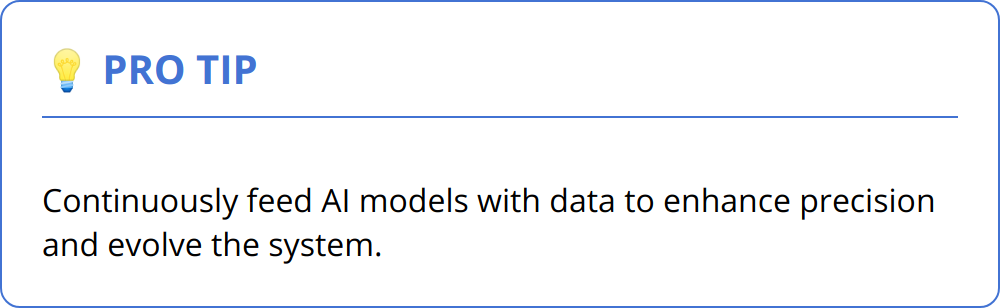
Artificial Intelligence breathes new life into image analysis by bringing speed and scalability. It does the heavy lifting that human eyes can’t sustain, processing thousands of images in the blink of an eye. What’s more, as AI models are continuously fed data, they evolve, becoming more precise with each iteration. AI doesn’t tire; it improves.
Practical Applications Across Industries
Image recognition isn’t confined to a single sector; its versatility sees it being applied from healthcare, where it aids in early disease detection, to agriculture, where it monitors crop health, and even retail, optimizing inventory management through automated stock images analysis. But it’s not enough to just implement these systems. Companies must be strategic. That means identifying where the technology can make the most significant impact, training models with industry-specific data, and integrating the system within existing workflows to truly see its benefits realized.
When deploying AI image recognition, you should consider these practical points:
-
Begin with a clear problem statement. What do you need image recognition to solve in your operations?
-
Use datasets that are representative of real-world scenarios your system will encounter.
-
Optimize models not just for accuracy but also for quick inference times, especially if used in real-time applications.
-
Regularly retrain the models with new data to keep the system at peak performance.
For example, the retail industry is using image recognition to provide a seamless visual search experience, allowing customers to find products by simply snapping a photo. On the other hand, the automotive sector uses this technology for advanced driver assistance systems, increasing vehicle safety through real-time hazard detection on the road.
To boil it down, success in AI image recognition is about marrying solid technical understanding with strategic, goal-oriented application. It’s about making smart decisions in data curation, being meticulous in model training, and ensuring the end solution aligns with the user’s needs. That’s how industries across the board are not just using AI, but using it effectively.
Implementing AI Image Recognition
When launching AI image recognition into your workflow, precise data annotation is not just helpful—it’s absolutely necessary. Picture this: every image that a model interprets is like a puzzle piece. When pieces are incorrectly labeled, the final picture ends up distorted. So, make sure your data is marked accurately to set your AI on the right track from the start.
Next, let’s talk diversity—in your data, that is. A model trained on a limited set of images will have just that—a limited understanding. If it hasn’t seen a wide variety of skin tones, for instance, it could misidentify people in the real world. That’s a big no-no. So, build your datasets to reflect the vibrant diversity of the global population.
Let’s not forget about continuous training and model optimization. AI doesn’t just ‘set it and forget it’. It needs to keep learning to stay sharp—sort of like how a chef sharpens their knives. When something changes in your dataset or the real world, your model should adapt. Keep feeding it new, relevant data and tweak its algorithms. That’s how you keep your AI image recognition system top-notch.

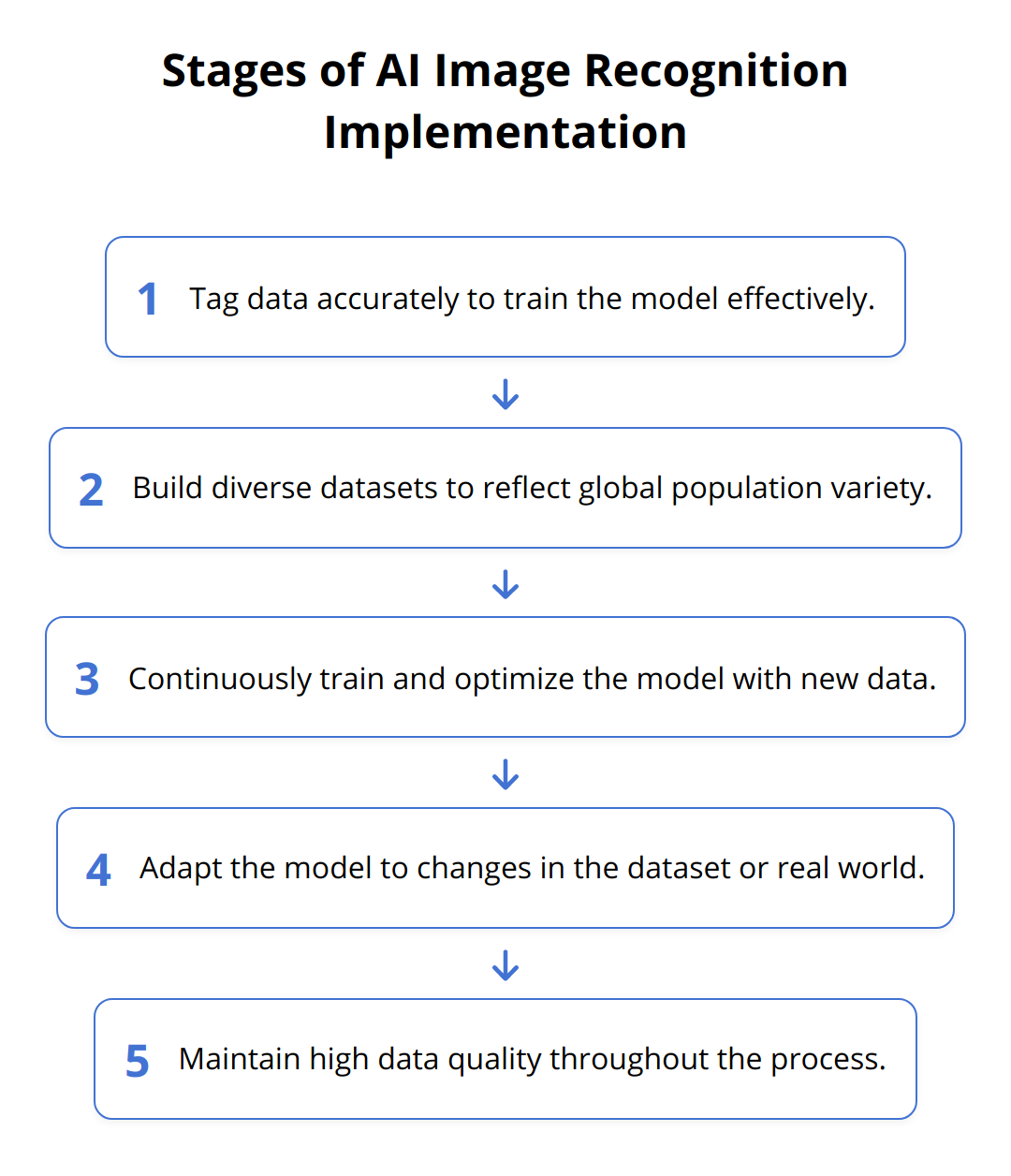
Here are quick bullet points to ensure you’re on the right track:
-
Tag your data accurately and comprehensively to train your model effectively.
-
Assemble datasets that truly represent the world your AI will operate in.
-
Keep your model in learning mode, improving continually with updated data.
Remember, whether you’re in retail or robotics, the key to a successful AI image recognition system lies in the specificity of your approach and rigorous adherence to data quality throughout the entire process. Implement these practices, and you’re on the path to unlocking the power of AI in your business.
And when you’re ready to take a deeper look into visual trends, exploring what’s around the corner in the realm of artificial intelligence and image recognition, visual search trends can provide you with insights into the future of this exciting technology.
Maximizing AI Efficiency

Achieving the ideal balance between speed and precision in AI-powered image recognition is pivotal for businesses that rely on this technology. It’s a fine line to walk, and the methods you choose can make or break the performance of your system. Here are practical insights to guide you in selecting the right tools and refining your processes for real-time decision making.
Selecting the Optimal Algorithms
Not all algorithms are created equal. For image recognition tasks, CNNs are the gold standard. However, within CNN architectures, there are variations like AlexNet, VGGNet, and ResNet. Each comes with its nuances and is suited for different complexity levels of image processing tasks.
Go for AlexNet or SqueezeNet for simpler applications; these are lighter and faster. But when dealing with more complex image datasets, VGGNet or ResNet can offer deeper layers for better accuracy. Always consider the trade-off between computational cost and accuracy. Your choice should align with the operational demands and resources at your disposal – efficiency is key.
Balancing Act Between Speed and Precision
In the fast-paced digital world, both speed and accuracy are vital. Here’s how to maintain this crucial balance:
-
Utilize smaller network architectures or apply network compression techniques for faster processing without a significant drop in accuracy.
-
Employ parallel processing techniques to speed up computation, especially if working with large-scale image datasets.
-
Consider hybrid models that use both traditional algorithms and neural networks to maximize efficiency.
Remember, an efficient AI system is one that not only recognizes images quickly but also does so with high reliability.
Real-time Processing Must-haves
Real-time processing is an absolute game-changer for industries needing instantaneous results, like security or autonomous driving. To integrate real-time capabilities:
-
Opt for edge computing, allowing for swift processing directly on devices, reducing latency considerably.
-
Leverage dedicated hardware like GPUs or TPUs to accelerate model inferences.
-
Streamline your model for quick deployment by using model quantization, which reduces the precision of the numbers involved in computations without compromising accuracy too much.
Here are some actionables to get you started:
-
Test various CNNs to find the best fit for your specific needs.
-
Prioritize model optimization for your application’s balance of speed and accuracy.
-
Regularly update your models with new data to prevent stagnation and performance drops.
By putting these practices into action, you’ll be well-equipped to build an AI image recognition system that doesn’t just work, but excels in real-world applications. The aim is not just to compete but to set the standard in your industry.
In the realm of AI, actions speak louder than words. For those ready to progress their strategies further, AI marketing tips provide additional guidance on strategic use of AI, enhancing not only image recognition but your overall business intelligence as well.
Final Thoughts
Embracing best practices in AI-powered image recognition is fundamental for achieving reliable and efficient results. The meticulous approach of continually refining algorithms, validating accurate datasets, and applying precision in annotation lays the groundwork for a robust AI image recognition system. Looking ahead, the trajectory of AI in image analysis is poised for remarkable advancements.
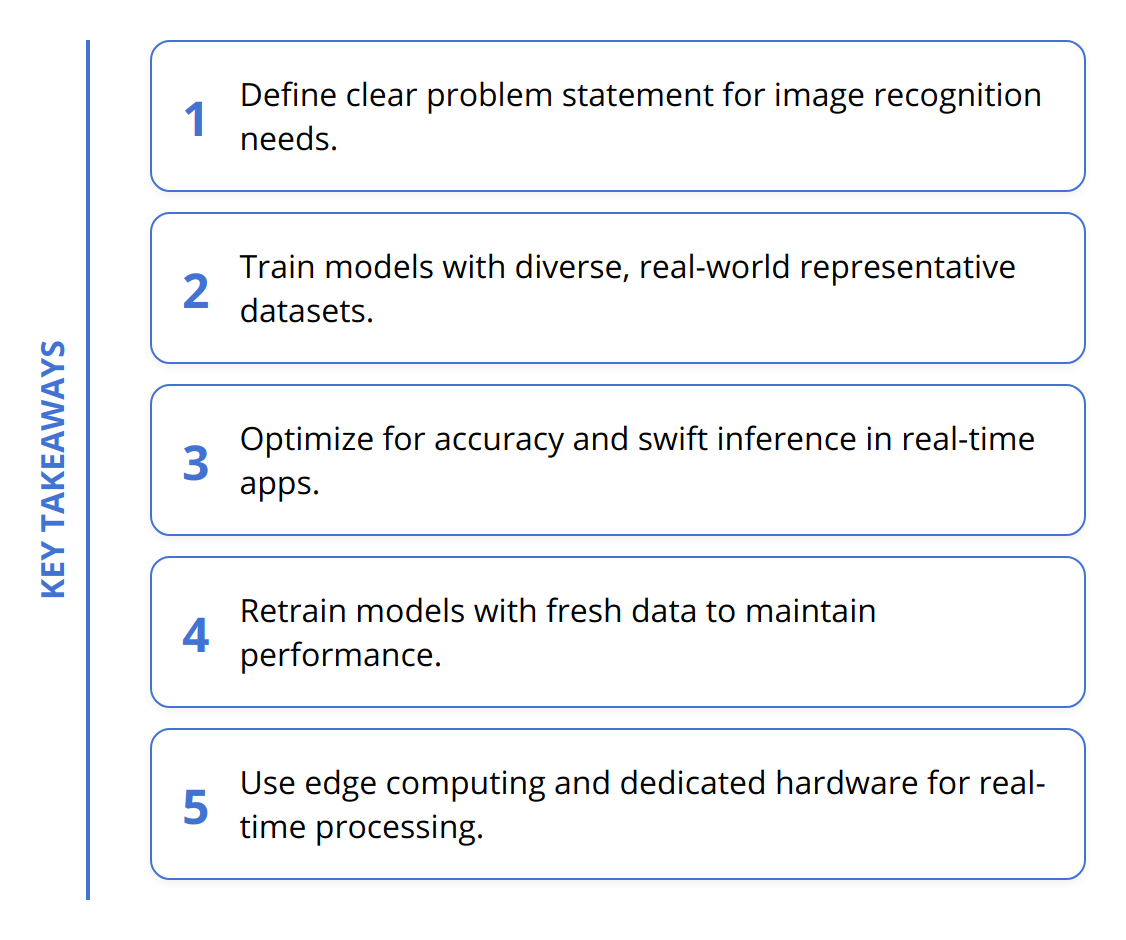
The future of AI in image recognition is poised to enhance the capabilities of various sectors by leaps and bounds. We at Emplibot anticipate groundbreaking progress in real-time processing, accuracy, and the ability to tackle complex visual tasks. This progression will likely pave the way for innovative applications that have yet to be conceived.
As technology evolves, it’s critical to foster the adoption of AI image recognition technology. Businesses that adopt AI will find themselves at the forefront, leveraging the power of advanced image analysis to drive efficiency and competitive edge. Emplibot stands ready to support this journey, offering a platform that seamlessly integrates with your existing systems, making the leap into AI as smooth as possible.
Implementing AI need not be an overwhelming conquest. With tools like Emplibot, businesses can effortlessly enhance their online presence. Emplibot’s automatic blog content capabilities, including keyword research and image integration, ensure that your site remains a topical authority with minimal manual input. This leaves more time to focus on core business strategies and the implementation of AI technologies like image recognition.
The importance of AI image recognition cannot be overstated, and its adoption is a testament to a company’s commitment to innovation and improvement. Here’s how your business can stay ahead:
-
Keep data quality and diversity non-negotiable in your AI strategy.
-
Continuously update and optimize models for improved performance.
-
Seek solutions like Emplibot for an agile and intelligent online presence.
In conclusion, the synergy between best practices in AI image recognition and strategic adoption in business processes is key. By welcoming these technological advancements, companies can elevate operations, enhance customer experiences, and ultimately drive growth. The future is bright for AI in image analysis, and for those who harness its full potential, the rewards are significant.