Machine learning is transforming how content reaches its intended audience, making personalization more accurate than ever before. We at Emplibot believe that leveraging this technology can significantly elevate your content strategy. Understanding its basics and implementing it effectively are key to unlocking personalized experiences for your users. This guide aims to offer practical insights and examples to help you achieve just that.
Contents
ToggleHow Machine Learning Powers Personalization
Machine learning has rapidly become a cornerstone of content personalization, offering capabilities that go far beyond traditional analytics and manual content creation. It presents a dynamic method for tailoring content to individual preferences, behaviors, and context in real-time.
The Foundation of Machine Learning in Personalization
At its core, machine learning analyzes vast amounts of data to identify patterns, preferences, and behaviors. It continuously learns from interactions, enabling the delivery of content that resonates on a personal level with users. This is not just about recommending products or services but creating a user experience that feels uniquely tailored to each individual.
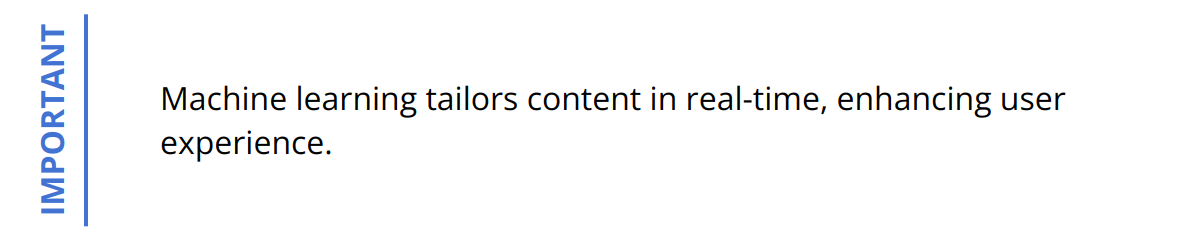
The Critical Role of Personalization
In today’s digital ecosystem, users are bombarded with an overwhelming amount of content daily. Personalization is not a luxury—it’s an expectation. Sites that fail to offer a personalized experience risk losing engagement, loyalty, and ultimately, revenue. A recent study showed that 80% of consumers are more likely to purchase from brands that provide personalized experiences.
Enhancing Content with Machine Learning
Machine learning elevates personalization by turning data into actionable insights. Here are some practical ways it enhances content strategy:
-
Predictive Analysis: Machine learning can predict user behavior, allowing content creators to craft messages that meet users’ needs before they even express them.
-
Dynamic Content Creation: With machine learning, content can dynamically adapt based on real-time user interactions, ensuring relevance at every touchpoint.
-
Segmentation at Scale: Machine learning algorithms can segment audiences with precision, enabling content creators to target specific groups with highly relevant content.
-
Continuous Optimization: Machine learning doesn’t just set and forget. It continuously refines its algorithms based on new data, leading to steadily improved personalization over time.
For practical insights into leveraging AI for content creation, visiting our post on enhancing content creation provides actionable tips and strategies.
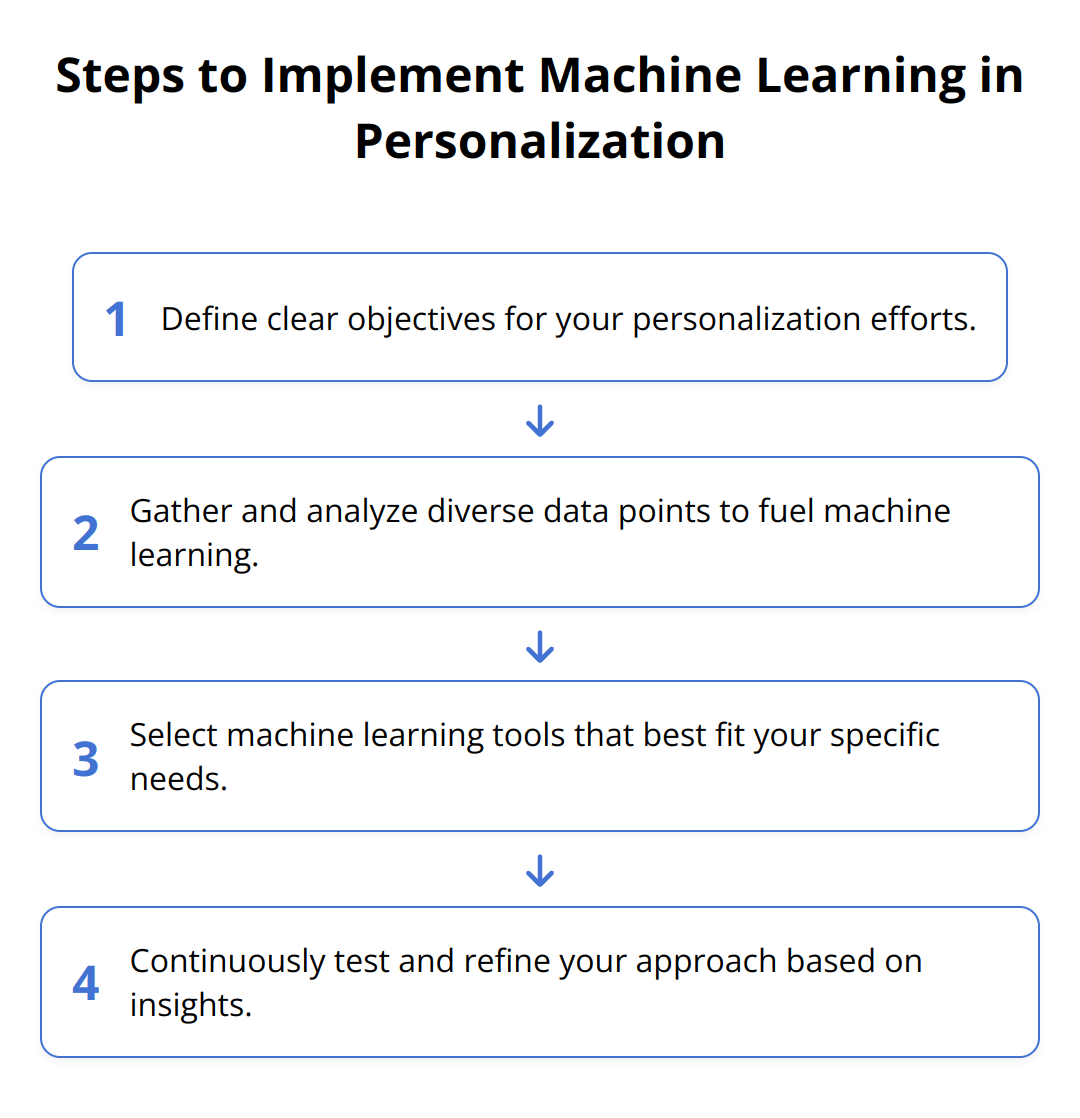
Tips for Implementing Machine Learning
-
Start with Clear Objectives: Define what success looks like for your personalization efforts. Is it increased engagement, higher conversion rates, or improved customer satisfaction?
-
Gather and Analyze Data: Collect data across various touchpoints. Machine learning relies on quality data to produce accurate insights.
-
Choose the Right Tools: Not all machine learning solutions are created equal. Select tools that align with your specific needs and integrate well with your existing technology stack.
-
Test and Learn: Implement A/B testing to understand what works best for your audience. Use these insights to continuously refine your approach.
By understanding the basics of machine learning and its significance in today’s content-driven world, businesses can harness its power to deliver unparalleled personalized experiences. The key is to leverage data effectively, continuously learn from interactions, and remain adaptable in your strategies. This approach will not only meet but exceed user expectations, fostering loyalty and driving success in the digital age.
Implementing ML in Content Strategies
Effective implementation of machine learning (ML) in content strategies hinges on systematic data collection, careful tool selection, and seamless integration into content creation and distribution processes. These elements work together to transform data into highly personalized user experiences.
Systematic Data Collection and Analysis
The foundation of any ML-driven personalization effort is high-quality data. Gathering comprehensive data sets from user interactions across all digital touchpoints is paramount. This includes website visits, email engagement, social media interactions, and even customer service inquiries.
Analyzing this data effectively requires a focus on identifying patterns that signal user preferences and behaviors. For example, if data shows that users frequently abandon their cart on an e-commerce site, content strategies might include personalized cart abandonment emails or targeted discounts to encourage completion of the purchase.
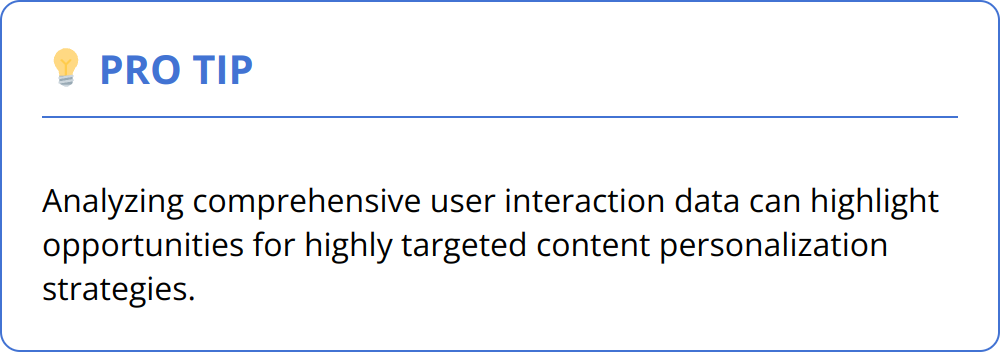
Selecting the Right Machine Learning Tools
Choosing the right ML tools is critical for the success of personalized content strategies. The market offers a plethora of options, but the best choice depends on specific business needs, existing tech stacks, and the level of customization required.
For businesses just starting with ML, platforms offering comprehensive analytics, user segmentation, and automated content recommendations might be the best fit. Tools that seamlessly integrate with existing content management systems and analytics platforms allow for a smoother transition into ML-driven personalization.
For more on this, see our piece on choosing AI tools.
Integrating ML into Content Creation and Distribution
Integrating ML into the content creation and distribution workflow enables real-time personalization and dynamic content adaptation. This means using ML insights to guide content topics, formats, and distribution channels for different audience segments.
For instance, insights derived from ML analysis could indicate that short-form video content shared on social media platforms performs well with a younger demographic, guiding the content creation team to prioritize these formats and channels.
Moreover, ML can optimize content distribution by determining the best times to publish or send emails to maximize engagement. Advanced algorithms can even predict the likelihood of users engaging with different content types, adjusting distribution strategies accordingly.
Key Takeaways:
-
Comprehensive data collection across digital touchpoints is essential.
-
The right ML tools should align with business needs and integrate with current systems.
-
Real-time personalization requires seamless integration of ML into content workflows.
By focusing on these areas, businesses can leverage ML to deliver content that not only meets but anticipates user needs, driving engagement and loyalty in the process. The journey toward ML-driven personalization is ongoing, with continuous learning and adaptation at its core.
Successful ML Content Personalization Examples
Leveraging Machine Learning (ML) for personalized content has set a new benchmark in engaging audiences effectively. Here, we dive into concrete examples demonstrating the power of ML in personalizing user experiences, notably in e-commerce, email marketing, and content suggestions.
E-commerce Recommendations
Online shopping platforms have revolutionized the way we discover products. Using ML algorithms, e-commerce sites can analyze a user’s browsing history, purchase patterns, and even the time spent on specific products. This data enables the delivery of precise product recommendations, significantly enhancing the shopping experience. For instance, Amazon’s recommendation system contributes to 35% of its total sales, highlighting the effectiveness of personalized recommendations in boosting revenue. Implementing similar ML-driven recommendation systems can lead to:
-
Increased customer satisfaction due to relevant suggestions.
-
Higher conversion rates with tailored offers.
-
Enhanced loyalty as customers experience a more personalized journey.

Personalized Email Marketing Campaigns
Email marketing remains a powerful tool for reaching out to customers. However, the one-size-fits-all approach is obsolete. Personalized email campaigns, driven by ML algorithms, analyze individual user actions, such as which emails they open, the links they click, and the products they browse. This analysis helps in segmenting the audience and delivering content that meets their interests. Companies leveraging personalized emails have seen up to 760% increase in email revenue. Strategies include:
-
Dynamic content insertion based on user preferences.
-
Optimized send times for each recipient to increase open rates.
-
Automated triggers based on user behavior, like browsing without buying.
For actionable advice on creating effective campaigns, our post on AI email optimization offers detailed strategies.
Customized News Feeds and Content Suggestions
Social media platforms and news aggregators heavily rely on ML to customize content feeds. By analyzing user interactions, such as likes, shares, and the time spent on articles, ML models can curate feeds that reflect each user’s interests. This level of personalization increases user engagement, keeps people on the platform longer, and enhances content discovery. Netflix, for example, saved $1 billion in 2017 through its personalized recommendation system by increasing user engagement. For content creators and platforms, this means:
-
Developing a deeper understanding of audience segments.
-
Delivering more relevant and engaging content that users value.
-
Increasing platform stickiness and reducing churn rates.
These examples showcase the transformative impact of ML in content personalization. By focusing on these strategies, businesses can offer exceptional personalized experiences, thereby increasing user satisfaction, engagement, and loyalty. The key lies in collecting detailed data, choosing the right ML tools, and continuously refining the personalization algorithms to keep pace with evolving user expectations and behaviors.
Wrapping Up
Machine Learning (ML) has indeed reshaped the landscape of content personalization, making it more dynamic, relevant, and engaging for users across various platforms. By leveraging the right tools and strategies, businesses can significantly enhance user experience, leading to increased engagement and loyalty. The ability of ML to analyze and predict user behavior offers a powerful means to deliver content that resonates personally with each user, thus setting new standards for digital interactions.
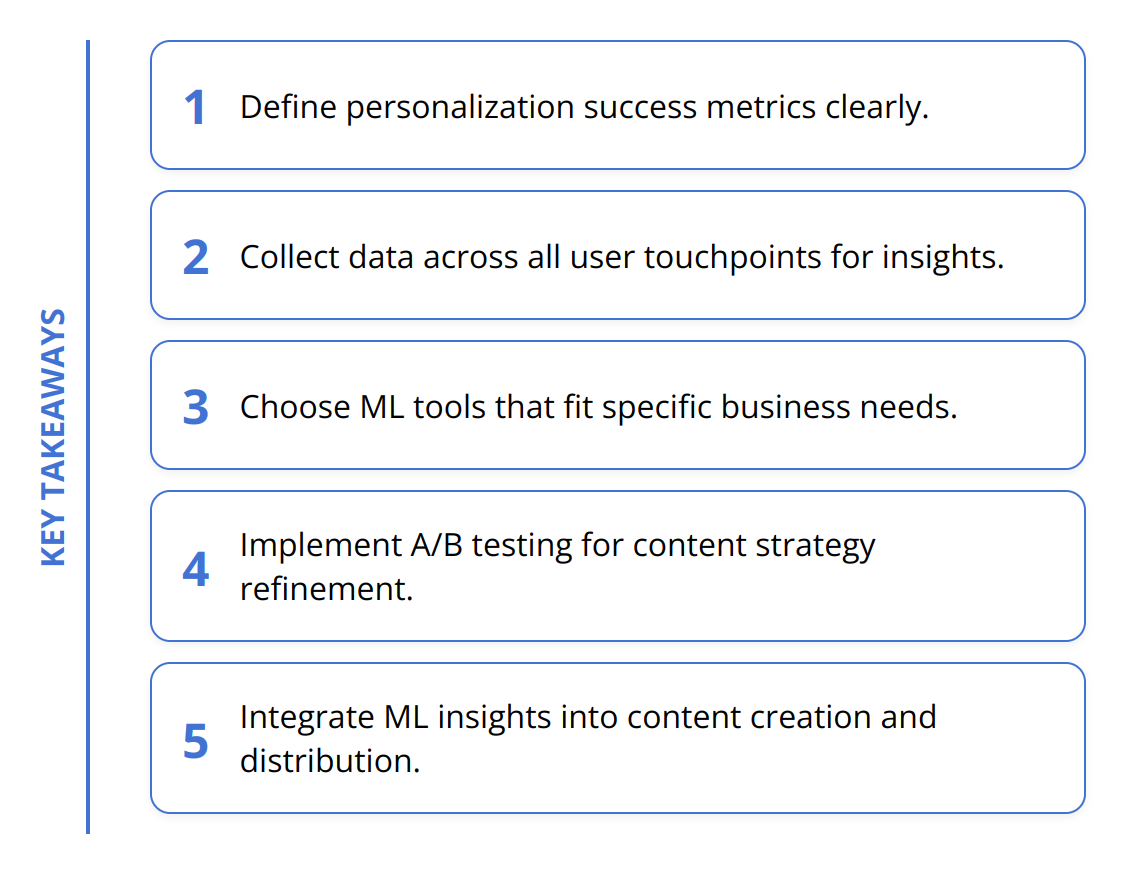
Looking ahead, the future of personalized content appears promising, with advancements in artificial intelligence and machine learning technologies paving the way for even more sophisticated personalization tactics. We’re likely to see a sharper focus on predictive personalization, where content doesn’t just react to user actions but anticipates needs and preferences before they’re explicitly expressed. The integration of voice and visual search could also add new dimensions to how content is personalized and consumed, making user interactions more natural and intuitive.
The landscape of ML in content personalization is ever-evolving, and staying abreast of these trends will be key to leveraging its full potential. Experimentation is vital. Testing different ML strategies and tools will help uncover what works best for your audience, providing invaluable insights into crafting more impactful and personalized content experiences.
Key Takeaways:
-
ML dramatically improves content relevance and user engagement.
-
Predictive personalization and advanced analytics are game-changers.
-
Continuous experimentation and adaptation are essential.
We at Emplibot are committed to making the journey towards more personalized and engaging content simpler and more effective. By automating the creation of SEO-friendly articles, including keyword research, images, and internal linking, we help businesses to enhance their digital presence effortlessly. Utilizing tools like Emplibot can streamline your content strategy, allowing you to focus on leveraging ML for personalization while we take care of the content creation process. Dive deeper into intelligent content strategies on Emplibot’s blog.
In this age of information overload, making your content stand out through personalization is no longer just an option—it’s a necessity. Machine learning offers a powerful toolkit for achieving this, enabling businesses to connect with their audience on a deeper level. As we look forward, the possibilities for personalized content are limitless, limited only by our willingness to experiment, learn, and evolve our strategies in tandem with technological advancements.